Distributional preferences in larger groups: keeping up with the Joneses and keeping track of the tails
What is this research about and why did you do it?
We want to investigate how individuals weigh income gaps between themselves and others in particular positions in a societal income distribution. This is crucial to understand how individuals form their fairness considerations and preferences for redistribution, as we know that people care about inequality both in absolute and in relative terms.
How did you answer this question?
We conduct a series of experiments via MechanicalTurk, in which subjects choose between two income distributions, each with seven (or more) individuals, with hypothetical incomes that aim to approximate the actual distribution of income in the U.S. In contrast to prior work, our design allows us to flexibly capture the particular distributional concerns of subjects.
What did you find?
Consistent with standard maximin (Rawlsian) preferences, subjects select distributions in which the bottom individual’s income is higher (but show little regard for lower incomes above the bottom ranking). In contrast to standard models, however, we find that subjects select distributions that lower the top individual’s income, but not other high incomes. Finally, we provide evidence of “locally competitive” preferences—in most experimental sessions, subjects select distributions that lower the income of the individual directly above them, while the income of the individual two positions above has little effect on subjects’ decisions. Our findings suggest that theories of inequality aversion should be adapted to account for individuals’ aversion to “topmost” and “local” disadvantageous inequality.
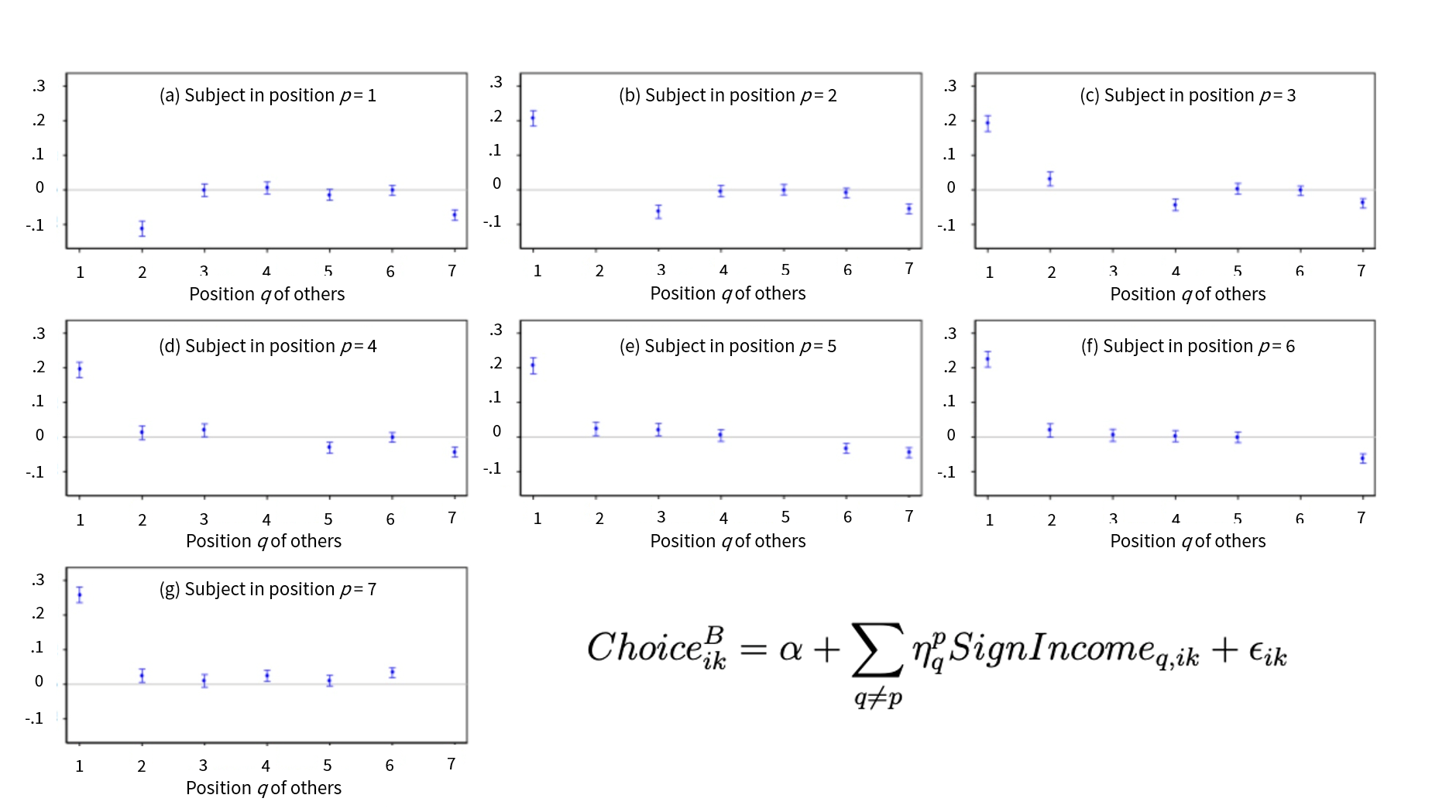
Effect of higher income in position q on subject’s propensity to choose distribution B over A, separately by position p of subject herself. The coefficients plotted for each graph are generated by performing the regression specified in equation 5 (see image below).The estimation in each case is for all decisions k made by subject i in which she was assigned a given position p in the income distribution. The plotted coefficients tell us whether subjects are more or less likely to choose a distribution that is favorable to position q when subjects are themselves in position p. The 95-percent confidence intervals plotted are based on standard errors clustered by subject.
As expected, regardless of assigned rank, for all p > 1,the coefficient for the person in position q=1 is large and positive, indicating that subjects in all positions put considerable weight on raising the income of the least well off individual (recall, this is not defined for p = 1). We similarly observe that for all p < 7, the coefficient for q=7 is negative across all panels, indicating a general desire to “soak the rich.” The only other case for which we observe a significant deviation from zero across all panels is for the position directly above the subject’s own. In every panel, the “one above” coefficient is negative and significantly different from zero at the five-percent level. No other coefficient in positions two through six is significant across all panels, regardless of its position relative to the subject.
What implications does this have for the research on wealth concentration or economic inequality?
This work highlights the importance of considering heterogeneity in the weight that individuals assign to relative income gaps with respect to different parts of the income distribution when trying to assess the determinants of distributional preferences. Different individuals might be supportive or averse to policies benefiting or taxing individuals that are relatively far or close to them in the income distribution. For example, workers earning just above the minimum wage might be less supportive of minimum wage raises relative to workers that earn slightly more, because for the former group the “local competition” effect is at play, and they might feel as they are losing out relative to the workers just below them that benefit from the wage increase. On the other hand, for the latter group, the increase is less salient as the people that would benefit from the minimum wage are not their immediate reference group.
What are the next steps in your agenda?
We want to better understand the determinants of attitudes toward income inequality for the U.S. overall, as well as for more intimate groups, e.g. attitudes towards pay inequality within companies or organizations. We also want to further explore what is the relevant comparison group individuals compare themselves to when forming attitudes towards inequality.
Citation
This paper can be cited as follows: Fisman, R., Kuziemko, I., and Vannutelli, S. (2021). "Distributional Preferences in Larger Groups: Keeping up with the Joneses and Keeping Track of the Tails." Journal of the European Economic Association, 19(2), pp. 1407-1438.