Predictably unequal? The effect of machine learning on credit markets
What is this research about and why did you do it?
The use of machine learning in credit allocation should allow lenders to better extend credit, but the shift from traditional to machine learning lending models may have important distributional effects for consumers. Our study analyzes the effect of machine learning on mortgage lending in the US. It finds that machine learning would offer lower rates to racial groups who already benefited from advantageous rates under the traditional model thus exacerbating distributional effects, but it would also benefit disadvantaged groups by enabling them to obtain a mortgage in the first place.
How did you answer this question?
We build simple theoretical frameworks to better understand the issues involved, and empirically estimate the likely impacts using a large administrative dataset from the US mortgage market, comprising about 10 million mortgage loans made between 2009 and 2013. In this setting, we compare the predictions that a hypothetical lender would make when using traditional statistics (e.g. standard Logit models) to those when using supervised machine learning techniques such as the Random Forest and XGBoost.
What did you find?
Figure 1 shows one of our key results. On the horizontal axis is the change in the log predicted default probability as lenders move from traditional technology (“Logit”) to machine learning (“Random Forest”). On the vertical is the cumulative share of borrowers from each racial group that experience a given level of change. Borrowers to the left of the solid vertical line are “winners” who are classed as less risky by the more sophisticated algorithm than by the traditional model. About 65% of White Non-Hispanic and Asian borrowers win, compared with about 50% of Black and Hispanic borrowers. The gains from new technology are therefore skewed in favour of racial groups that already enjoy socio-economic advantages.
The paper goes further in showing that these effects are driven mostly by the flexibility of new technology, as opposed to by machine learning algorithms’ ability to essentially proxy for, or triangulate, borrowers’ race. We also decompose the equilibrium effects of changes in statistical technology in a model of competitive credit provision.
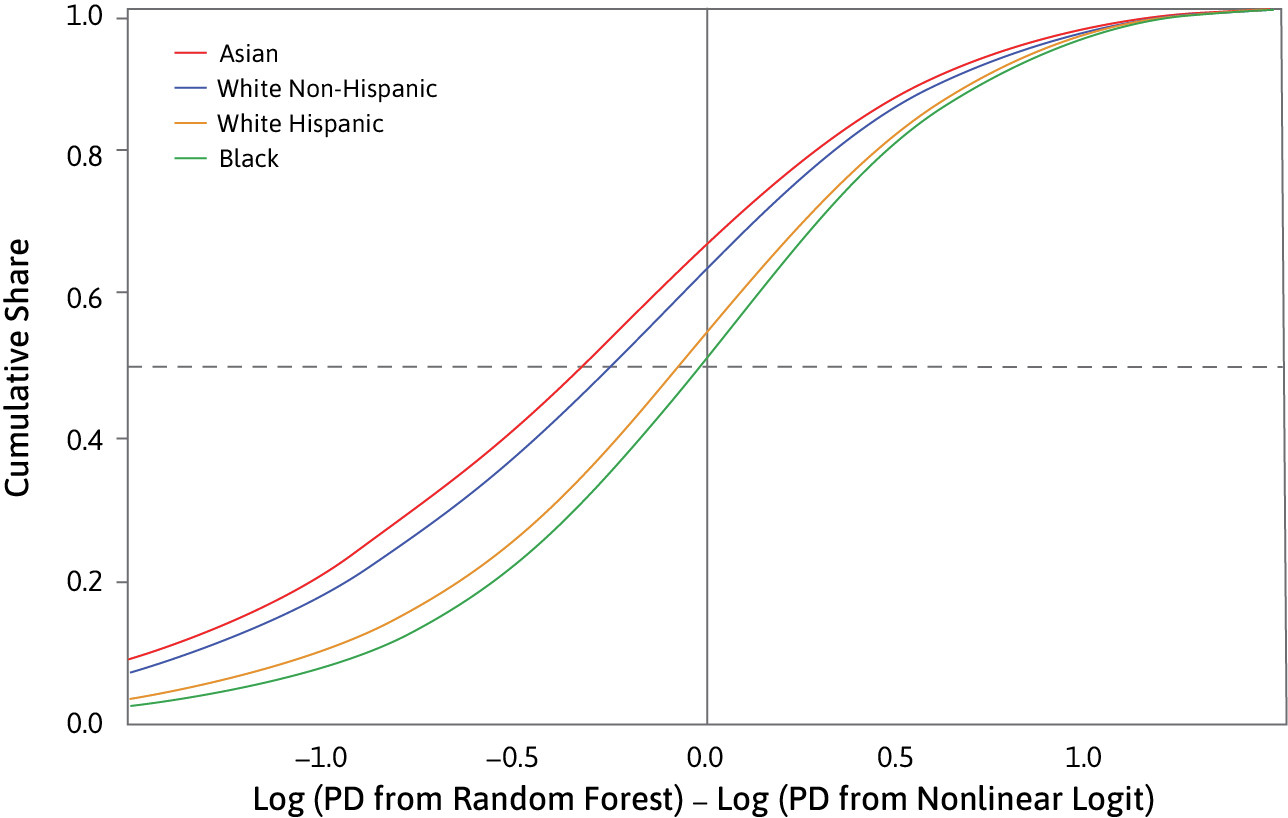
What implications does this have for the study on wealth concentration or economic inequality?
Our research has taken a first step towards a deeper understanding of the problems associated with the widespread adoption of new machine learning technologies. In the US mortgage market, we find that concerns about unequal effects are indeed valid. Perhaps more importantly, however, we lay out a framework for assessing and decomposing such effects, which can be applied beyond our dataset.
What are the next steps in your agenda?
Further exploration of how technological innovation in finance can affect inequality.
Citation
This paper can be cited as follows: Fuster, A., Goldsmith-Pinkham, P., Ramadorai, T., and Walther, A. (2022) "Predictably unequal? The effects of machine learning on credit markets." The Journal of Finance, 77(1), pp. 5-47.