Who should get vaccinated? Individualized allocation of vaccines over SIR network
What is this research about and why did you do it?
Allocation of scarce resources among individuals interacting in a social network is an important policy decision in many fields, including medicine, education, and economics. Our research was motivated by the problem of how to allocate vaccines in the Covid-19 pandemic among heterogeneous individuals to control the spread of the disease and protect the vulnerable. Devising an optimal allocation rule when there are spillovers over the network remains a challenging open question. This research develops a method to identify an individualized allocation of vaccines among individuals who interact in a social network with an epidemiological model of disease transmission (heterogeneous Susceptible-Infectious-Recovered (SIR) model).
How did you answer this question?
Exploiting social network data containing individual demographic characteristics and health status, we develop a procedure to estimate an individualized vaccine allocation policy when supply is limited. We introduce a heterogeneous-interacted-SIR model to model the spillover effects of an infectious disease and show that the welfare objective function of the vaccine allocation problem satisfies a certain decreasing-returns property (known as sub-modularity). Building on these findings, we propose an algorithm that sequentially allocates a vaccine to an individual in the network who is most influential for improving social welfare and show guaranteed performance in terms of a societal public health criterion. We also show the ‘greedy algorithm’ method can accommodate exogenous capacity constraints across different demographic groups, which policymakers commonly face in reality.
What did you find?
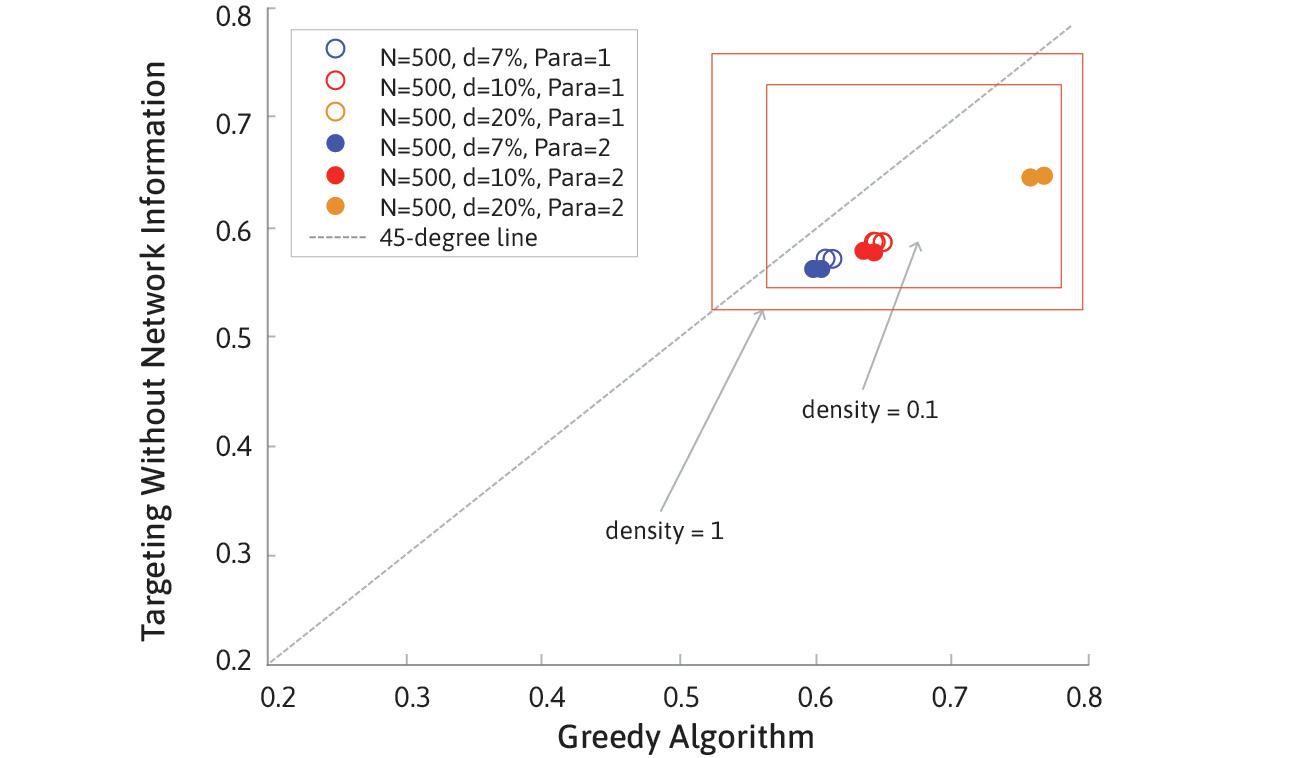
The numbers on each axis reflect the value of welfare (the sum of probabilities of being healthy in the second period). For different parameters (network size and density) used in the simulation, welfare is higher when the ‘greedy algorithm’ is used to allocate the vaccine rather than targeting the vaccine without using the network information. This outcome is shown by the observations lying to the right of the 45-degree line.
Our numerical study shows the effectiveness of individualized assignment of vaccines considering spillovers, and the importance of targeting using network information. Compared with a random assignment policy and a targeting policy without network information, our assignment policy improves welfare by 4%–12%. This result is insensitive to the values of the SIR parameters and the size and density of the network. It suggests that even under the state of strict containment policy in the early phase of the pandemic, where the density of the network is maintained at a relatively low level, the cost of ignoring spillovers is significant for vaccine allocation.
What implications does this have for the research on wealth concentration or economic inequality?
This work highlights the importance of network information in the individualized vaccine allocation problem. Ignoring network information leads to an inefficient allocation of resources. Such inefficiency increases dramatically as the number of vaccines and the size of the network expand. General policy implications of our work are:
- collecting network information is extremely valuable for designing treatment assignment policy,
- a computationally attractive greedy algorithm is useful to devise an effective policy.
What are the next steps in your agenda?
We want to extend this analysis to assignment policies that run over multiple allocation periods. We also want to analyze what type of fairness constraints can be incorporated in the individualized treatment allocation problems over the network.
Citation
This paper can be cited as follows: Kitagawa, T. and Wang, G. (2023) 'Who Should Get Vaccinated? Individualized Allocation of Vaccines Over SIR Network.' Journal of Econometrics, 232(1): pp. 109-131.